
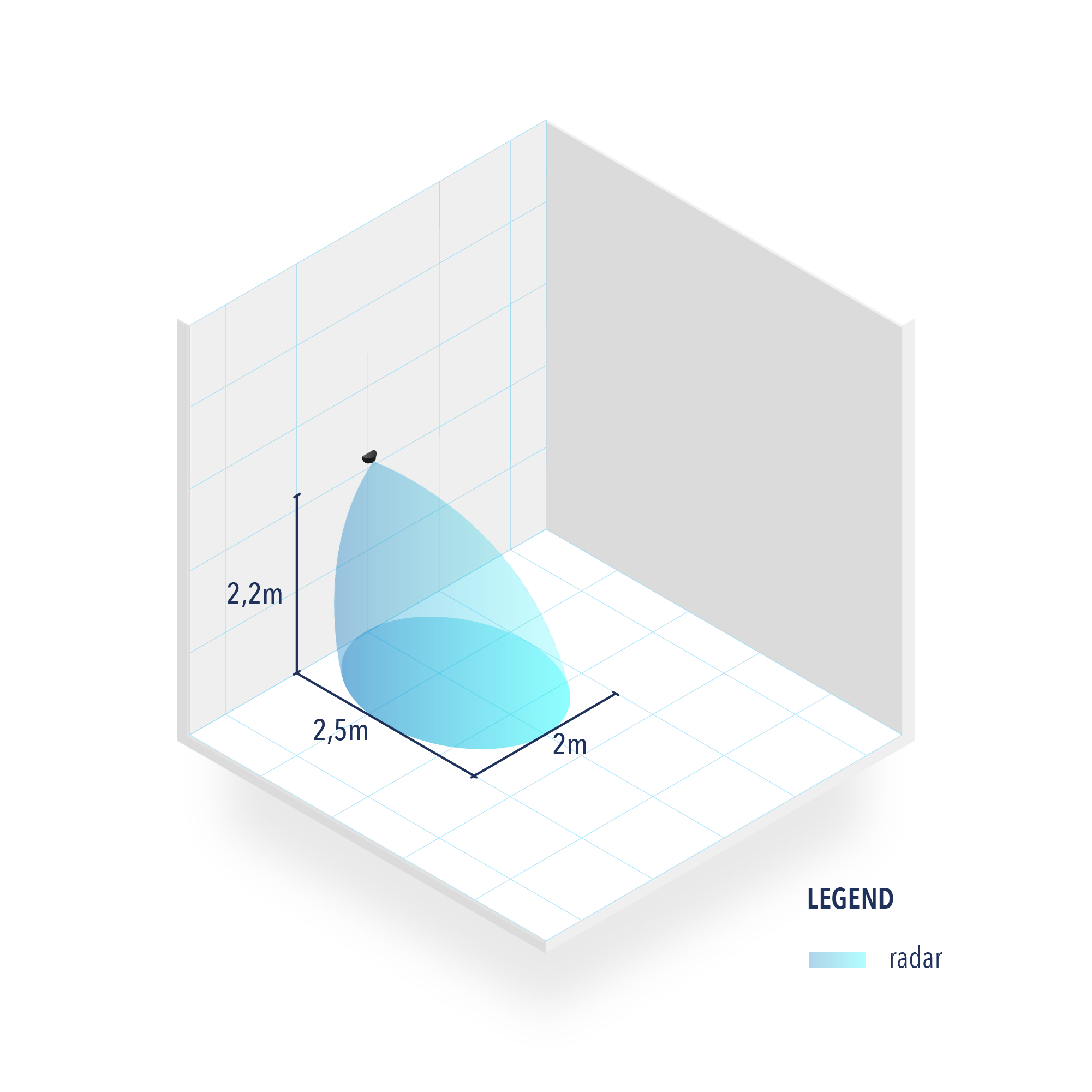
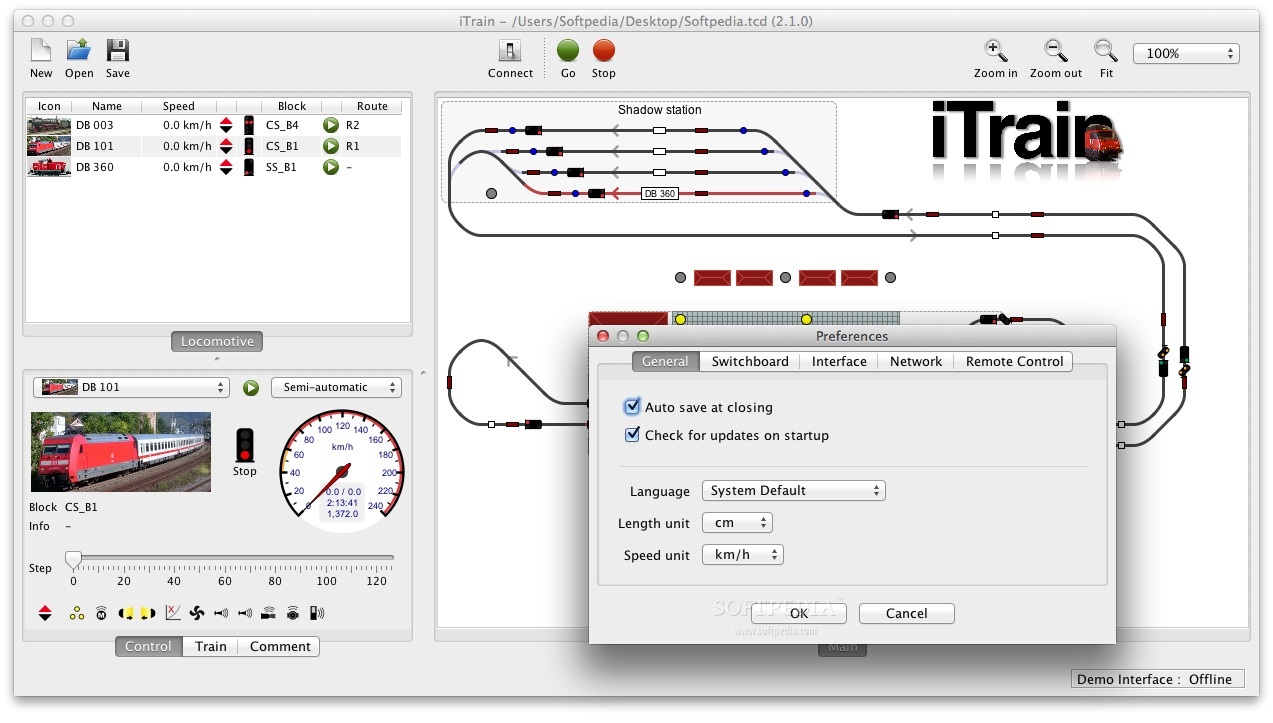
In our work, we use machine learning (ML) techniques in order to investigate how chemotaxis-based decision making can be learned and performed in a viscous environment. From an evolutionary point of view, it remains elusive how motility and chemotactic patterns evolved together, bearing in mind that both different prokaryotic and eukaryotic cells with diverse self-propulsion mechanisms developed surprisingly similar chemotactic machinery ( 14, 16, 17). In contrast to bacteria, many eukaryotic cells such as Dictyostelium ( 12, 13), leukocytes ( 14), or cancer cells ( 15) are able to perform chemotaxis by adapting their migration direction in accordance with the CG by spatial sensing with membrane receptors. Some bacteria follow more diverse chemotactic strategies, which can be related to their specific propulsion mechanisms ( 11). This process is mediated by a time-dependent response function which suppresses tumbling when swimming upward chemical gradients (CGs) ( 5, 8– 10). Bacteria use temporal information of chemical field concentrations to perform chemotaxis. An important example is the run-and-tumble motion of various bacteria such as Escherichia coli ( 5, 6) or of the algae Chlamydomonas ( 7). In order to search for nutrients, oxygen, or light, they have developed mechanisms to change their shape and, hence, their swimming direction abruptly. Microorganisms possess a huge variety of different self-propulsion strategies in order to actively swim through viscous fluids such as water, which is realized by performing periodic nonreciprocal deformations of their body shape ( 1– 4). These findings are of relevance for intracellular biochemical sensing mechanisms of single cells or for the simple nervous system of small multicellular organisms such as Caenorhabditis elegans. Our work demonstrates that the evolution of a simple and interpretable internal decision-making machinery coupled to the environment allows navigation in diverse chemical landscapes. By introducing noisy signal transmission in the neural network, the well-known biased run-and-tumble motion emerges. These networks control the shape deformations of the microswimmers and allow them to navigate in static and complex time-dependent chemical environments. Using the genetic algorithm NeuroEvolution of Augmenting Topologies, surprisingly simple neural networks evolve. We present two methods to measure chemical gradients, spatial and temporal sensing, as known for swimming mammalian cells and bacteria, respectively.

As an internal decision-making machinery, we use artificial neural networks, which control the motion of the microswimmer. Here, we propose a computational model, which features autonomous shape adaptation of microswimmers moving in one dimension toward high field concentrations. In order to perform chemotaxis (i.e., to move toward and to stay at high concentrations of nutrients), they adapt their swimming gaits in a nontrivial manner.

Various microorganisms and some mammalian cells are able to swim in viscous fluids by performing nonreciprocal body deformations, such as rotating attached flagella or by distorting their entire body.
